Publications
DreamUDF: Generating Unsigned Distance Fields from A Single Image
Yu-Tao Liu, Xuan Gao, Weikai Chen, Jie Yang, Xiaoxu Meng, Bo Yang, Lin Gao
SIGGRAPH Asia 2024 (ACM Transactions on Graphics)
"a 3D generative model that can generate shapes with arbitrary topologies, including open surfaces"
Yu-Tao Liu, Xuan Gao, Weikai Chen, Jie Yang, Xiaoxu Meng, Bo Yang, Lin Gao
SIGGRAPH Asia 2024 (ACM Transactions on Graphics)
"a 3D generative model that can generate shapes with arbitrary topologies, including open surfaces"
- paper
-
abstract
Recent advances in diffusion models and neural implicit surfaces have shown promising progress in generating 3D models. However, existing generative frameworks are limited to closed surfaces, failing to cope with a wide range of commonly seen shapes that have open boundaries. In this work, we present DreamUDF, a novel framework for generating high-quality 3D objects with arbitrary topologies from a single image. To address the challenge of generating proper topology given sparse and ambiguous observations, we propose to incorporate both the data priors from a multi-view diffusion model and the geometry priors brought by an unsiged distance field (UDF) reconstructor. In particular, we leverage a joint framework that consists of 1) a generative module that produces a neural radiance field that provides photo-realistic renderings from the arbitrary view; and 2) a reconstructive module that distills the learnable radiance field into surfaces with arbitrary topologies. We further introduce a field coupler that bridges the radiance field and UDF under an novel optimization scheme. This allows the two modules to mutually boost each other during training. Extensive experiments and evaluations demonstrate that DreamUDF achieves high-quality reconstruction and robust 3D generation on both closed and open surfaces with arbitrary topologies, compared to the previous works.
GarVerseLOD: High-Fidelity 3D Garment Reconstruction from a Single In-the-Wild Image using a Dataset with Levels of Details
Zhongjin Luo, Haolin Liu, Chenghong Li, Wanghao Du, Zirong Jin, Yinyu Nie, Weikai Chen, Xiaoguang Han
SIGGRAPH Asia 2024 (ACM Transactions on Graphics)
"first 3D garment dataset with levels of details for enhancing generalizability"
Zhongjin Luo, Haolin Liu, Chenghong Li, Wanghao Du, Zirong Jin, Yinyu Nie, Weikai Chen, Xiaoguang Han
SIGGRAPH Asia 2024 (ACM Transactions on Graphics)
"first 3D garment dataset with levels of details for enhancing generalizability"
- paper
-
abstract
Neural implicit functions have brought impressive advances to the state-of-the-art of clothed human digitization from multiple or even single images. However, despite the progress, current arts still have difficulty generalizing to unseen images with complex cloth deformation and body poses. In this work, we present GarVerseLOD, a new dataset and framework that paves the way to achieving unprecedented robustness in high-fidelity 3D garment reconstruction from a single unconstrained image. Inspired by the recent success of large generative models, we believe that one key to addressing the generalization challenge lies in the quantity and quality of 3D garment data. Towards this end, GarVerseLOD collects 6000 high-quality cloth models with fine-grained geometry details manually created by professional artists. In addition to the scale of training data, we observe that having disentangled granularities of geometry can play an important role in boosting the generalization capability and inference accuracy of the learned model. We hence craft GarVerseLOD as a hierarchical dataset with evels of details (LOD), spanning from detail-free stylized shape to pose-blended garment with pixel-aligned details. This allows us to make this highly under-constrained problem tractable by factorizing the inference into easier tasks, each narrowed down with smaller searching space. To ensure GarVerseLOD can generalize well to in-the-wild images, we propose a novel labeling paradigm based on conditional diffusion models to generate extensive paired images for each garment model with high photorealism. We evaluate our method on a massive amount of in-the-wild images. Experimental results demonstrate that GarVerseLOD can generate standalone garment pieces with significantly better quality than prior approaches while being robust against a large variation of pose, illumination, occlusion, and deformation. Both code and dataset will be released.
MarvelOVD: Marrying Object Recognition and Vision-Language Models for Robust Open-Vocabulary Object Detection
Kuo Wang, Lechao Cheng, Weikai Chen, Pingping Zhang, Liang Lin, Fan Zhou, Guanbin Li
ECCV 2024
"visual detector can be a strong auxiliary guidance to VLM tasks"
Kuo Wang, Lechao Cheng, Weikai Chen, Pingping Zhang, Liang Lin, Fan Zhou, Guanbin Li
ECCV 2024
"visual detector can be a strong auxiliary guidance to VLM tasks"
- project page
- paper
- code
-
abstract
Learning from pseudo-labels that generated with VLMs (Vision Language Models) has been shown as a promising solution to assist open vocabulary detection (OVD) in recent studies. However, due to the domain gap between VLM and vision-detection tasks, pseudo-labels produced by the VLMs are prone to be noisy, while the training design of the detector further amplifies the bias. In this work, we investigate the root cause of VLMs’ biased prediction under the OVD context. Our observations lead to a simple yet effective paradigm, coded MarvelOVD, that generates significantly better training targets and optimizes the learning procedure in an online manner by marrying the capability of the detector with the vision-language model. Our key insight is that the detector itself can act as a strong auxiliary guidance to accommodate VLM’s inability of understanding both the “background” and the context of a proposal within the image. Based on it, we greatly purify the noisy pseudo-labels via Online Mining and propose Adaptive Reweighting to effectively suppress the biased training boxes that are not well aligned with the target object. In addition, we also identify a neglected “base-novel-conflict” problem and introduce stratified label assignments to prevent it. Extensive experiments on COCO and LVIS datasets demonstrate that our method outperforms the other state-of-the-arts by significant margins. Codes are available at https://github.com/wkfdb/MarvelOVD.
OVER-NAV: Elevating Iterative Vision-and-Language Navigation with Open-Vocabulary Detection and StructurEd Representation
Ganlong Zhao, Guanbin Li, Weikai Chen, Yizhou Yu
CVPR 2024
"first VLN using open-vocabulary detection and structured representation"
Ganlong Zhao, Guanbin Li, Weikai Chen, Yizhou Yu
CVPR 2024
"first VLN using open-vocabulary detection and structured representation"
- paper
-
abstract
Recent advances in Iterative Vision-and-Language Navigation (IVLN) introduce a more meaningful and practical paradigm of VLN by maintaining the agent's memory across tours of scenes. Although the long-term memory aligns better with the persistent nature of the VLN task, it poses more challenges on how to utilize the highly unstructured navigation memory with extremely sparse supervision. Towards this end, we propose OVER-NAV, which aims to go over and beyond the current arts of IVLN techniques. In particular, we propose to incorporate LLMs and open-vocabulary detectors to distill key information and establish correspondence between multi-modal signals. Such a mechanism introduces reliable cross-modal supervision and enables on-the-fly generalization to unseen scenes without the need of extra annotation and re-training. To fully exploit the interpreted navigation data, we further introduce a structured representation, coded Omnigraph, to effectively integrate multi-modal information along the tour. Accompanied with a novel omnigraph fusion mechanism, OVER-NAV is able to extract the most relevant knowledge from omnigraph for a more accurate navigating action. In addition, OVER-NAV seamlessly supports both discrete and continuous environments under a unified framework. We demonstrate the superiority of OVER-NAV in extensive experiments.
SENSE: Self-Evolving Learning for Self-Supervised Monocular Depth Estimation
Guanbin Li, Ricong Huang, Haofeng Li, Zunzhi You, Weikai Chen
IEEE Transactions on Image Processing (TIP) 2023
"self-supervised monocular depth estimation"
Guanbin Li, Ricong Huang, Haofeng Li, Zunzhi You, Weikai Chen
IEEE Transactions on Image Processing (TIP) 2023
"self-supervised monocular depth estimation"
- paper
-
abstract
Self-supervised depth estimation methods can achieve competitive performance using only unlabeled monocular videos, but they suffer from the uncertainty of jointly learning depth and pose without any ground truths of both tasks. Supervised framework provides robust and superior performance but is limited by the scope of the labeled data. In this paper, we introduce SENSE, a novel learning paradigm for self-supervised monocular depth estimation that progressively evolves the prediction result using supervised learning, but without requiring labeled data. The key contribution of our approach stems from the novel use of the pseudo labels – the noisy depth estimation from the self-supervised methods. We surprisingly find that a fully supervised depth estimation network trained using the pseudo labels can produce even better results than its “ground truth”. To push the envelope further, we then evolve the self-supervised backbone by replacing its depth estimation branch with that fully supervised network. Based on this idea, we devise a comprehensive training pipeline that alternatively enhances the two key branches (depth and pose estimation) of the self-supervised backbone network. Our proposed approach can effectively ease the difficulty of multi-task training in self-supervised depth estimation. Experimental results have shown that our proposed approach achieves state-of-the-art results on the KITTI dataset.
Get3DHuman: Lifting StyleGAN-Human into a 3D Generative Model using Pixel-aligned Reconstruction Priors
Zhangyang Xiong, Di Kang, Derong Jin, Weikai Chen, Linchao Bao, Shuguang Cui, Xiaoguang Han
ICCV 2023
"generative model for 3D human that combines both 2D and 3D priors"
Zhangyang Xiong, Di Kang, Derong Jin, Weikai Chen, Linchao Bao, Shuguang Cui, Xiaoguang Han
ICCV 2023
"generative model for 3D human that combines both 2D and 3D priors"
- project page
- paper
- code
-
abstract
Fast generation of high-quality 3D digital humans is important to a vast number of applications ranging from entertainment to professional concerns. Recent advances in differentiable rendering have enabled the training of 3D generative models without requiring 3D ground truths. However, the quality of the generated 3D humans still has much room to improve in terms of both fidelity and diversity. In this paper, we present Get3DHuman, a novel 3D human framework that can significantly boost the realism and diversity of the generated outcomes by only using a limited budget of 3D ground-truth data. Our key observation is that the 3D generator can profit from human-related priors learned through 2D human generators and 3D reconstructors. Specifically, we bridge the latent space of Get3DHuman with that of StyleGAN-Human via a specially-designed prior network, where the input latent code is mapped to the shape and texture feature volumes spanned by the pixel-aligned 3D reconstructor The outcomes of the prior network are then leveraged as the supervisory signals for the main generator network. To ensure effective training, we further propose three tailored losses applied to the generated feature volumes and the intermediate feature maps. Extensive experiments demonstrate that Get3DHuman greatly outperforms the other state-of-the-art approaches and can support a wide range of applications including shape interpolation, shape re-texturing, and single-view reconstruction through latent inversion.
RankMatch: Fostering Confidence and Consistency in Learning with Noisy Labels
Ziyi Zhang, Weikai Chen, Chaowei Fang, Zhen Li, Lechao Chen, Liang Lin, Guanbin Li
ICCV 2023
"new paradigm for learning with noisy labels"
Ziyi Zhang, Weikai Chen, Chaowei Fang, Zhen Li, Lechao Chen, Liang Lin, Guanbin Li
ICCV 2023
"new paradigm for learning with noisy labels"
- paper
-
abstract
Learning with noisy labels (LNL) is one of the most important and challenging problems in weakly-supervised learning. Recent advances adopt the sample selection strategy to mitigate the interference of noisy labels and use small-loss criteria to select clean samples. However, the one-dimensional loss is an over-simplified metric that fails to accommodate the complex feature landscape of various samples, and, hence, is prone to introduce classification errors during sample selection. In this paper, we propose RankMatch, a novel LNL framework that investigates additional dimensions of confidence and consistency in order to combat noisy labels. Confidence-wise, we propose a novel sample selection strategy based on confidence representation voting instead of the widely-used small-loss criterion. This new strategy is capable of increasing sample selection quantity without sacrificing labeling accuracy. Consistency-wise, instead of the widely adopted feature distance metric for measuring the consistency of inner-class samples, we advocate that the rank of principal features is a much more robust indicator. Based on this metric, we propose rank contrastive loss, which strengthens the consistency of similar samples regardless of their labels and facilitates feature representation learning. Experimental results on noisy versions of CIFAR-10, CIFAR-100, Clothing1M, and WebVision have validated the superiority of our approach over existing state-of-the-art methods.
NeUDF: Leaning Neural Unsigned Distance Fields with Volume Rendering
Yu-Tao Liu, Li Wang, Jie Yang, Weikai Chen, Xiaoxu Meng, Bo Yang, Lin Gao
CVPR 2023
"NeuS for unsigned distance field"
Yu-Tao Liu, Li Wang, Jie Yang, Weikai Chen, Xiaoxu Meng, Bo Yang, Lin Gao
CVPR 2023
"NeuS for unsigned distance field"
- project page
- paper
- code
-
abstract
Multi-view shape reconstruction has achieved impressive progresses thanks to the latest advances in neural implicit rendering. However, existing methods based on signed distance function (SDF) are limited to closed surfaces, failing to reconstruct a wide range of real-world objects that contain open-surface structures. In this work, we introduce a new neural rendering framework, coded NeUDF, that can reconstruct surfaces with arbitrary topologies solely from multi-view supervision. To gain the flexibility of representing arbitrary surfaces, NeUDF leverages the unsigned distance function (UDF) as surface representation. While a naive extension of SDF-based neural renderer cannot scale to UDF, we propose two new formulations of weight function specially tailored for UDF-based volume rendering. Furthermore, to cope with open surface rendering, where the in/out test is no longer valid, we present a dedicated normal regularization strategy to resolve the surface orientation ambiguity. We extensively evaluate our method over a number of challenging datasets, including two typical open surface datasets MGN and Deep Fashion 3D. Experimental results demonstrate that NeUDF can significantly outperform the state-of-the-art methods in the task of multi-view surface reconstruction, especially for the complex shapes with open boundaries.
NeAT: Learning Neural Implicit Surfaces with Arbitrary Topologies from Multi-view Images
Xiaoxu Meng, Weikai Chen, Bo Yang
CVPR 2023
"NeuS for 3-Pole signed distance field (3PSDF)"
Xiaoxu Meng, Weikai Chen, Bo Yang
CVPR 2023
"NeuS for 3-Pole signed distance field (3PSDF)"
- project page
- paper
- code
- data
-
abstract
Recent progress in neural implicit functions has set new state-of-the-art in reconstructing high-fidelity 3D shapes from a collection of images. However, these approaches are limited to closed surfaces as they require the surface to be represented by a signed distance field. In this paper, we propose NeAT, a new neural rendering framework that can learn implicit surfaces with arbitrary topologies from multi-view images. In particular, NeAT represents the 3D surface as a level set of a signed distance function (SDF) with a validity branch for estimating the surface existence probability at the query positions. We also develop a novel neural volume rendering method, which uses SDF and validity to calculate the volume opacity and avoids rendering points with low validity. NeAT supports easy field-to-mesh conversion using the classic Marching Cubes algorithm. Extensive experiments on DTU, MGN, and Deep Fashion 3D datasets indicate that our approach is able to faithfully reconstruct both watertight and non-watertight surfaces. In particular, NeAT significantly outperforms the state-of-the-art methods in the task of open surface reconstruction both quantitatively and qualitatively.
NerVE: Neural Volumetric Edges for Parametric Curve Extraction from Point Cloud
Xiangyu Zhu, Dong Du, Weikai Chen, Zhiyou Zhao, Yinyu Nie, Xiaoguang Han
CVPR 2023
"a neural volumetric representation for learning parametric curves"
Xiangyu Zhu, Dong Du, Weikai Chen, Zhiyou Zhao, Yinyu Nie, Xiaoguang Han
CVPR 2023
"a neural volumetric representation for learning parametric curves"
- project page
- paper
- video
-
abstract
Extracting parametric edge curves from point clouds is a fundamental problem in 3D vision and geometry processing. Existing approaches mainly rely on keypoint detection, a challenging procedure that tends to generate noisy output, making the subsequent edge extraction error-prone. To address this issue, we propose to directly detect structured edges to circumvent the limitations of the previous point-wise methods. We achieve this goal by presenting NerVE, a novel neural volumetric edge representation that can be easily learned through a volumetric learning framework. NerVE can be seamlessly converted to a versatile piece-wise linear (PWL) curve representation, enabling a unified strategy for learning all types of free-form curves. Furthermore, as NerVE encodes rich structural information, we show that edge extraction based on NerVE can be reduced to a simple graph search problem. After converting NerVE to the PWL representation, parametric curves can be obtained via off-the-shelf spline fitting algorithms. We evaluate our method on the challenging ABC dataset. We show that a simple network based on NerVE can already outperform the previous state-of-the-art methods by a great margin
Divide and Adapt: Active Domain Adaptation via Customized Learning
Duojun Huang, Jichang Li, Weikai Chen, Junshi Huang, Zhenhua Chai, Guanbin Li
CVPR 2023 (Highlight)
"a versatile framework for source-free domain adaptation"
Duojun Huang, Jichang Li, Weikai Chen, Junshi Huang, Zhenhua Chai, Guanbin Li
CVPR 2023 (Highlight)
"a versatile framework for source-free domain adaptation"
- paper
-
abstract
We investigate a practical domain adaptation task, called source-free domain adaptation (SFUDA), where the source-pretrained model is adapted to the target domain without access to the source data. Existing techniques mainly leverage self-supervised pseudo labeling to achieve class-wise global alignment [1] or rely on local structure extraction that encourages feature consistency among neighborhoods [2]. While impressive progress has been made, both lines of methods have their own drawbacks - the "global" approach is sensitive to noisy labels while the "local" counterpart suffers from source bias. In this paper, we present Divide and Contrast (DaC), a new paradigm for SFUDA that strives to connect the good ends of both worlds while bypassing their limitations. Based on the prediction confidence of the source model, DaC divides the target data into source-like and target-specific samples, where either group of samples is treated with tailored goals under an adaptive contrastive learning framework. Specifically, the source-like samples are utilized for learning global class clustering thanks to their relatively clean labels. The more noisy target-specific data are harnessed at the instance level for learning the intrinsic local structures. We further align the source-like domain with the target-specific samples using a memory bank-based Maximum Mean Discrepancy (MMD) loss to reduce the distribution mismatch. Extensive experiments on VisDA, Office-Home, and the more challenging DomainNet have verified the superior performance of DaC over current state-of-the-art approaches.
IMPLICITPCA: Implicitly-Proxied Parametric Encoding for Collision-Aware Garment Reconstruction
Lan Chen, Jie Yang, Hongbo Fu, Xiaoxu Meng, Weikai Chen, Bo Yang, Lin Gao
CVM 2023
"a parametric SDF network that handles collision detection and garment reconstruction"
Lan Chen, Jie Yang, Hongbo Fu, Xiaoxu Meng, Weikai Chen, Bo Yang, Lin Gao
CVM 2023
"a parametric SDF network that handles collision detection and garment reconstruction"
- paper
-
abstract
The emerging remote collaboration in a virtual environment calls for the need for high-fidelity 3D human reconstruction from single image.To deal with the challenges of cloth details and topologies, parametric models are widely used as explicit priors. While they often lack of fine details from the image. Neural implicit approaches generate accurate details but are typically limited to closed surfaces.In addition, physically correct reconstructions, e.g. collision-free, is crucial but often ignored in prior works.We present ImplicitPCA, a parametric SDF network that closely couples parametric encoding with implicit functions, to enjoy the fine details brought by implicit reconstruction while maintaining correct open surfaces.We introduce a fast collision-aware regression network to ensure physically-correct estimation.During inference, an iterative routine is applied to align the garment to the 2D landmarks and fit with the collision-aware cloth SDF.The experiments on the public dataset and in-the-wild images demonstrate our outperformance.
HSDF: Hybrid Sign and Distance Field for Modeling Surfaces with Arbitrary Topologies
Li Wang, Jie Yang, Weikai Chen, Xiaoxu Meng, Bo Yang, Jintao Li, Lin Gao
NeurIPS 2022, TVCG 2024
"a learnable implicit representation for modeling both closed and open surfaces"
Li Wang, Jie Yang, Weikai Chen, Xiaoxu Meng, Bo Yang, Jintao Li, Lin Gao
NeurIPS 2022, TVCG 2024
"a learnable implicit representation for modeling both closed and open surfaces"
- paper
-
abstract
Neural implicit function based on signed distance field (SDF) has achieved impressive progress in reconstructing 3D models with high fidelity. However, such approaches can only represent closed shapes. Recent works based on unsigned distance function (UDF) are proposed to handle both watertight and open surfaces. Nonetheless, as UDF is signless, its direct output is limited to point cloud, which imposes an additional challenge on extracting high-quality meshes from discrete points. To address this issue, we present a new learnable implicit representation, coded HSDF, that connects the good ends of SDF and UDF. In particular, HSDF is able to represent arbitrary topologies containing both closed and open surfaces while being compatible with existing iso-surface extraction techniques for easy field-to-mesh conversion. In addition to predicting a UDF, we propose to learn an additional sign field via a simple classifier. Unlike traditional SDF, HSDF is able to locate the surface of interest before level surface extraction by generating surface points following NDF. We are then able to obtain open surfaces via an adaptive meshing approach that only instantiates regions containing surface into a polygon mesh. We also propose HSDF-Net, a dedicated learning framework that factorizes the learning of HSDF into two easier problems. Experiments on multiple datasets show that HSDF outperforms state-of-the-art techniques both qualitatively and quantitatively.
Divide and Contrast: Source-free Domain Adaptation via Adaptive Contrastive Learning
Ziyi Zhang, Weikai Chen, Hui Cheng, Zhen Li, Siyuan Li, Liang Lin, Guanbin Li
NeurIPS 2022
"a new method for source-free domain adaptation"
Ziyi Zhang, Weikai Chen, Hui Cheng, Zhen Li, Siyuan Li, Liang Lin, Guanbin Li
NeurIPS 2022
"a new method for source-free domain adaptation"
- paper
- code
-
abstract
We investigate a practical domain adaptation task, called source-free domain adaptation (SFUDA), where the source pretrained model is adapted to the target domain without access to the source data. Existing techniques mainly leverage self-supervised pseudo-labeling to achieve class-wise global alignment [1] or rely on local structure extraction that encourages the feature consistency among neighborhoods [2]. While impressive progress has been made, both lines of methods have their own drawbacks – the “global” approach is sensitive to noisy labels while the “local” counterpart suffers from the source bias. In this paper, we present Divide and Contrast (DaC), a new paradigm for SFUDA that strives to connect the good ends of both worlds while bypassing their limitations. Based on the prediction confidence of the source model, DaC divides the target data into source-like and target-specific samples, where either group of samples is treated with tailored goals under an adaptive contrastive learning framework. Specifically, the source-like samples are utilized for learning global class clustering thanks to their relatively clean labels. The more noisy target-specific data are harnessed at the instance level for learning the intrinsic local structures. We further align the source-like domain with the target-specific samples using a memory bank-based Maximum Mean Discrepancy (MMD) loss to reduce the distribution mismatch. Extensive experiments on VisDA, Office-Home, and the more challenging DomainNet have verified the superior performance of DaC over current state-of-the-art approaches. The code is available at https://github.com/ZyeZhang/DaC.git.
3PSDF: Three-Pole Signed Distance Function for Learning Surfaces with Arbitrary Topologies
Weikai Chen, Cheng Lin, Weiyang Li, Bo Yang
CVPR 2022
"a new implicit representation that can represent non-watertight shapes"
Weikai Chen, Cheng Lin, Weiyang Li, Bo Yang
CVPR 2022
"a new implicit representation that can represent non-watertight shapes"
- project page
- paper
- code
-
abstract
Recent advances in learning 3D shapes using neural implicit functions have achieved impressive results by breaking the previous barrier of resolution and diversity for varying topologies. However, most of such approaches are limited to closed surfaces as they require the space to be divided into inside and outside. More recent works based on unsigned distance function have been proposed to handle complex geometry containing both the open and closed surfaces. Nonetheless, as their direct outputs are point clouds, robustly obtaining high-quality meshing results from discrete points remains an open question. We present a novel learnable implicit representation, called three-pole signed distance function (3PSDF), that can represent non-watertight 3D shapes with arbitrary topologies while supporting easy field-to-mesh conversion using the classic Marching Cubes algorithm. The key to our method is the introduction of a new sign, the NULL sign, in addition to the conventional in and out labels. The existence of the null sign could stop the formation of a closed isosurface derived from the bisector of the in/out regions. Further, we propose a dedicated learning framework to effectively learn 3PSDF without worrying about the vanishing gradient due to the null labels. Experimental results show that our approach outperforms the previous state-of-the-art methods in a wide range of benchmarks both quantitatively and qualitatively.
Dual Adversarial Adaptation for Cross-Device Real-World Image Super-Resolution
Xiaoqian Xu, Pengxu Wei, Weikai Chen, Mingzhi Mao, Liang Lin, Guanbin Li
CVPR 2022 (Oral Presentation)
"the first work studying cross-device domain adaptation for real-world image super-resolution"
Xiaoqian Xu, Pengxu Wei, Weikai Chen, Mingzhi Mao, Liang Lin, Guanbin Li
CVPR 2022 (Oral Presentation)
"the first work studying cross-device domain adaptation for real-world image super-resolution"
- paper
- code
-
abstract
Due to the sophisticated imaging process, an identical scene captured by different cameras could exhibit distinct imaging patterns, introducing distinct proficiency among the super-resolution (SR) models trained on images from different devices. In this paper, we investigate a novel and practical task coded cross-device SR, which strives to adapt a real-world SR model trained on the paired images captured by one camera to low-resolution (LR) images captured by arbitrary target devices. The proposed task is highly challenging due to the absence of paired data from various imaging devices. To address this issue, we propose an unsupervised domain adaptation mechanism for real-world SR, named Dual ADversarial Adaptation (DADA), which only requires LR images in the target domain with available real paired data from a source camera. DADA employs the Domain-Invariant Attention (DIA) module to establish the basis of target model training even without HR supervision. Furthermore, the dual framework of DADA facilitates an Inter-domain Adversarial Adaptation (InterAA) in one branch for two LR input images from two domains, and an Intra-domain Adversarial Adaptation (IntraAA) in two branches for an LR input image. InterAA and IntraAA together improve the model transferability from the source domain to the target. We empirically conduct experiments under six Real→Real adaptation settings among three different cameras, and achieve superior performance compared with existing state-of-the-art approaches. We also evaluate the proposed DADA to address the adaptation to the video camera, which presents a promising research topic to promote the wide applications of real-world super-resolution. Our source code is publicly available at https://github.com/lonelyhope/DADA.
Exemplar-based Pattern Synthesis with Implicit Periodic Field Network
Haiwei Chen, Jiayi Liu, Weikai Chen, Shichen Liu, Yajie Zhao
CVPR 2022
"a general method for synthesizing 2D images and 3D structures using implicit periodic function"
Haiwei Chen, Jiayi Liu, Weikai Chen, Shichen Liu, Yajie Zhao
CVPR 2022
"a general method for synthesizing 2D images and 3D structures using implicit periodic function"
- paper
- code
-
abstract
Synthesis of ergodic, stationary visual patterns is widely applicable in texturing, shape modeling, and digital content creation. The wide applicability of this technique thus requires the pattern synthesis approaches to be scalable, diverse, and authentic. In this paper, we propose an exemplar-based visual pattern synthesis framework that aims to model the inner statistics of visual patterns and generate new, versatile patterns that meet the aforementioned requirements. To this end, we propose an implicit network based on generative adversarial network (GAN) and periodic encoding, thus calling our network the Implicit Periodic Field Network (IPFN). The design of IPFN ensures scalability: the implicit formulation directly maps the input coordinates to features, which enables synthesis of arbitrary size and is computationally efficient for 3D shape synthesis. Learning with a periodic encoding scheme encourages diversity: the network is constrained to model the inner statistics of the exemplar based on spatial latent codes in a periodic field. Coupled with continuously designed GAN training procedures, IPFN is shown to synthesize tileable patterns with smooth transitions and local variations. Last but not least, thanks to both the adversarial training technique and the encoded Fourier features, IPFN learns high-frequency functions that produce authentic, high-quality results. To validate our approach, we present novel experimental results on various applications in 2D texture synthesis and 3D shape synthesis.
OctField: Hierarchical Implicit Functions for 3D Modeling
Jia-Heng Tang*, Weikai Chen*, Jie Yang, Bo Wang, Songrun Liu, Bo Yang, Lin Gao
NeurIPS 2021
"a new hierarchical implicit representation with its generative model for high-precision modeling with low cost"
Jia-Heng Tang*, Weikai Chen*, Jie Yang, Bo Wang, Songrun Liu, Bo Yang, Lin Gao
NeurIPS 2021
"a new hierarchical implicit representation with its generative model for high-precision modeling with low cost"
- project page
- paper
- code
-
abstract
Recent advances in localized implicit functions have enabled neural implicit representation to be scalable to large scenes. However, the regular subdivision of 3D space employed by these approaches fails to take into account the sparsity of the surface occupancy and the varying granularities of geometric details. As a result, its memory footprint grows cubically with the input volume, leading to a prohibitive computational cost even at a moderately dense decomposition. In this work, we present a learnable hierarchical implicit representation for 3D surfaces, coded OctField, that allows high-precision encoding of intricate surfaces with low memory and computational budget. The key to our approach is an adaptive decomposition of 3D scenes that only distributes local implicit functions around the surface of interest. We achieve this goal by introducing a hierarchical octree structure to adaptively subdivide the 3D space according to the surface occupancy and the richness of part geometry. As octree is discrete and non-differentiable, we further propose a novel hierarchical network that models the subdivision of octree cells as a probabilistic process and recursively encodes and decodes both octree structure and surface geometry in a differentiable manner. We demonstrate the value of OctField for a range of shape modeling and reconstruction tasks, showing superiority over alternative approaches.
Trash to Treasure: Harvesting OOD Data with Cross-Modal Matching for Open-Set Semi-Supervised Learning
Junkai Huang, Chaowei Fang, Weikai Chen, Zhenhua Chai, Xiaolin Wei, Pengxu Wei, Liang Lin, Guanbin Li
ICCV 2021
"a new framework for open-set semi-supervised learning that fully utilizes OOD data"
Junkai Huang, Chaowei Fang, Weikai Chen, Zhenhua Chai, Xiaolin Wei, Pengxu Wei, Liang Lin, Guanbin Li
ICCV 2021
"a new framework for open-set semi-supervised learning that fully utilizes OOD data"
- paper
-
abstract
Open-set semi-supervised learning (open-set SSL) investigates a challenging but practical scenario where out-of-distribution (OOD) samples are contained in the unlabeled data. While the mainstream technique seeks to completely filter out the OOD samples for semi-supervised learning (SSL), we propose a novel training mechanism that could effectively exploit the presence of OOD data for enhanced feature learning while avoiding its adverse impact on the SSL. We achieve this goal by first introducing a warm-up training that leverages all the unlabeled data, including both the in-distribution (ID) and OOD samples. Specifically, we perform a pretext task that enforces our feature extractor to obtain a high-level semantic understanding of the training images, leading to more discriminative features that can benefit the downstream tasks. Since the OOD samples are inevitably detrimental to SSL, we propose a novel cross-modal matching strategy to detect OOD samples. Instead of directly applying binary classification, we train the network to predict whether the data sample is matched to an assigned one-hot class label. The appeal of the proposed cross-modal matching over binary classification is the ability to generate a compatible feature space that aligns with the core classification task. Extensive experiments show that our approach substantially lifts the performance on open-set SSL and outperforms the state-of-the-art by a large margin.
Deep Optimized Priors for 3D Shape Modeling and Reconstruction
Mingyue Yang, Yuxin Wen, Weikai Chen, Yongwei Chen, Kui Jia
CVPR 2021
"a framework to improve the performance and generality of 3D shape prior"
Mingyue Yang, Yuxin Wen, Weikai Chen, Yongwei Chen, Kui Jia
CVPR 2021
"a framework to improve the performance and generality of 3D shape prior"
- project page
- paper
-
abstract
Many learning-based approaches have difficulty scaling to unseen data, as the generality of its learned prior is limited to the scale and variations of the training samples. This holds particularly true with 3D learning tasks, given the sparsity of 3D datasets available. We introduce a new learning framework for 3D modeling and reconstruction that greatly improves the generalization ability of a deep generator. Our approach strives to connect the good ends of both learning-based and optimization-based methods. In particular, unlike the common practice that fixes the pre-trained priors at test time, we propose to further optimize the learned prior and latent code according to the input physical measurements after the training. We show that the proposed strategy effectively breaks the barriers constrained by the pre-trained priors and could lead to high-quality adaptation to unseen data. We realize our framework using the implicit surface representation and validate the efficacy of our approach in a variety of challenging tasks that take highly sparse or collapsed observations as input. Experimental results show that our approach compares favorably with the state-of-the-art methods in terms of both generality and accuracy.
Equivariant Point Network for 3D Point Cloud Analysis
Haiwei Chen, Shichen Liu, Weikai Chen, Hao Li
CVPR 2021
"a new approach for extracting SE(3) equivariant feature for point clouds"
Haiwei Chen, Shichen Liu, Weikai Chen, Hao Li
CVPR 2021
"a new approach for extracting SE(3) equivariant feature for point clouds"
- paper
- code
-
abstract
Features that are equivariant to a larger group of symmetries have been shown to be more discriminative and powerful in recent studies. However, higher-order equivariant features often come with an exponentiallygrowing computational cost. Furthermore, it remains relatively less explored how rotation-equivariant features can be leveraged to tackle 3D shape alignment tasks. While many past approaches have been based on either nonequivariant or invariant descriptors to align 3D shapes, we argue that such tasks may benefit greatly from an equivariant framework. In this paper, we propose an effective and practical SE(3) (3D translation and rotation) equivariant network for point cloud analysis that addresses both problems. First, we present SE(3) separable point convolution, a novel framework that breaks down the 6D convolution into two separable convolutional operators alternatively performed in the 3D Euclidean and SO(3) spaces. This significantly reduces the computational cost without compromising the performance. Second, we introduce an attention layer to effectively harness the expressiveness of the equivariant features. While jointly trained with the network, the attention layer implicitly derives the intrinsic local frame in the feature space and generate attention vectors that can be integrated in different alignment tasks. We evaluate our approach through extensive studies and visual interpretations. The empirical results demonstrate that our proposed model outperforms strong baselines in a variety of benchmarks.
3DCaricShop: A Dataset and A Baseline Method for Single-view 3D Caricature Face Reconstruction
Yuda Qiu, Xiaojie Xu, Linteng Qiu, Yan Pan, Yushuang Wu, Weikai Chen, Xiaoguang Han
CVPR 2021
"a large scale dataset for 3D caricature"
Yuda Qiu, Xiaojie Xu, Linteng Qiu, Yan Pan, Yushuang Wu, Weikai Chen, Xiaoguang Han
CVPR 2021
"a large scale dataset for 3D caricature"
- project page
- paper
-
abstract
Caricature is an artistic representation that deliberately exaggerates the distinctive features of a human face to convey humor or sarcasm. However, reconstructing a 3D caricature from a 2D caricature image remains a challenging task, mostly due to the lack of data. We propose to fill this gap by introducing 3DCaricShop, the first large-scale 3D caricature dataset that contains 2000 high-quality diversified 3D caricatures manually crafted by professional artists. 3DCaricShop also provides rich annotations including a paired 2D caricature image, camera parameters, and 3D facial landmarks. To demonstrate the advantage of 3DCaricShop, we present a novel baseline approach for single-view 3D caricature reconstruction. To ensure a faithful reconstruction with plausible face deformations, we propose to connect the good ends of the detail-rich implicit functions and the parametric mesh representations. In particular, we first register a template mesh to the output of the implicit generator and iteratively project the registration result onto a pre-trained PCA space to resolve artifacts and self-intersections. To deal with the large deformation during non-rigid registration, we propose a novel view-collaborative graph convolution network (VC-GCN) to extract key points from the implicit mesh for accurate alignment. Our method is able to generate high-fidelity 3D caricature in a pre-defined mesh topology that is animation-ready. Extensive experiments have been conducted on 3DCaricShop to verify the significance of the database and the effectiveness of the proposed method. We will release 3DCaricShop upon publication.
Deep Fashion3D: A Dataset and Benchmark for 3D Garment Reconstruction from Single Images
Heming Zhu, Yu Cao, Hang Jin, Weikai Chen, Dong Du, Zhangye Wang, Shuguang Cui, and Xiaoguang Han
ECCV 2020 (Oral Presentation)
"a large scale dataset and benchmark for real 3D garments"
Heming Zhu, Yu Cao, Hang Jin, Weikai Chen, Dong Du, Zhangye Wang, Shuguang Cui, and Xiaoguang Han
ECCV 2020 (Oral Presentation)
"a large scale dataset and benchmark for real 3D garments"
- project page
- paper
-
abstract
High-fidelity clothing reconstruction is the key to achieving photorealism in a wide range of applications including human digitization, virtual try-on, etc. Recent advances in learning-based approaches have accomplished unprecedented accuracy in recovering unclothed human shape and pose from single images, thanks to the availability of powerful statistical models, e.g. SMPL [38], learned from a large number of body scans. In contrast, modeling and recovering clothed human and 3D garments remains notoriously difficult, mostly due to the lack of large-scale clothing models available for the research community. We propose to fill this gap by introducing Deep Fashion3D, the largest collection to date of 3D garment models, with the goal of establishing a novel benchmark and dataset for the evaluation of image-based garment reconstruction systems. Deep Fashion3D contains 2078 models reconstructed from real garments, which covers 10 different categories and 563 garment instances. It provides rich annotations including 3D feature lines, 3D body pose and the corresponded multi-view real images. In addition, each garment is randomly posed to enhance the variety of real clothing deformations. To demonstrate the advantage of Deep Fashion3D, we propose a novel baseline approach for single-view garment reconstruction, which leverages the merits of both mesh and implicit representations. A novel adaptable template is proposed to enable the learning of all types of clothing in a single network. Extensive experiments have been conducted on the proposed dataset to verify its significance and usefulness. We will make Deep Fashion3D publicly available upon publication
A General Differentiable Mesh Renderer for Image-based 3D Reasoning
Shichen Liu, Tianye Li, Weikai Chen*, and Hao Li
IEEE Transactions on Pattern Analysis and Machine Intelligence (TPAMI) 2020
"an extension of our ICCV'19 paper SoftRas"
Shichen Liu, Tianye Li, Weikai Chen*, and Hao Li
IEEE Transactions on Pattern Analysis and Machine Intelligence (TPAMI) 2020
"an extension of our ICCV'19 paper SoftRas"
- paper
-
abstract
Rendering bridges the gap between 2D vision and 3D scenes by simulating the physical process of image formation. By inverting such renderer, one can think of a learning approach to infer 3D information from 2D images. However, standard graphics renderers involve a fundamental step called rasterization, which prevents rendering to be differentiable. Unlike the state-of-the-art differentiable renderers, which only approximate the rendering gradient in the backpropagation, we propose a natually differentiable rendering framework that is able to (1) directly render colorized mesh using differentiable functions and (2) back-propagate efficient supervisions to mesh vertices and their attributes from various forms of image representations. The key to our framework is a novel formulation that views rendering as an aggregation function that fuses the probabilistic contributions of all mesh triangles with respect to the rendered pixels. Such formulation enables our framework to flow gradients to the occluded and distant vertices, which cannot be achieved by the previous state-of-the-arts. We show that by using the proposed renderer, one can achieve significant improvement in 3D unsupervised single-view reconstruction both qualitatively and quantitatively. Experiments also demonstrate that our approach can handle the challenging tasks in image-based shape fitting, which remain nontrivial to existing differentiable renders
Intuitive, Interactive Beard and Hair Synthesis with Generative Models
Kyle Olszewski, Duygu Ceylan, Jun Xing, Jose I. Echevarria, Zhili Chen, Weikai Chen, Hao Li
CVPR 2020 (Oral Presentation)
"deep learning powered interactive facial hair editing system"
Kyle Olszewski, Duygu Ceylan, Jun Xing, Jose I. Echevarria, Zhili Chen, Weikai Chen, Hao Li
CVPR 2020 (Oral Presentation)
"deep learning powered interactive facial hair editing system"
- paper
-
abstract
We present an interactive approach to synthesizing real-istic variations in facial hair in images, ranging from subtleedits to existing hair to the addition of complex and chal-lenging hair in images of clean-shaven subjects. To cir-cumvent the tedious and computationally expensive tasks ofmodeling, rendering and compositing the 3D geometry ofthe target hairstyle using the traditional graphics pipeline,we employ a neural network pipeline that synthesizes real-istic and detailed images of facial hair directly in the tar-get image in under one second. The synthesis is controlledby simple and sparse guide strokes from the user definingthe general structural and color properties of the targethairstyle. We qualitatively and quantitatively evaluate ourchosen method compared to several alternative approaches.We show compelling interactive editing results with a proto-type user interface that allows novice users to progressivelyrefine the generated image to match their desired hairstyle,and demonstrate that our approach also allows for flexibleand high-fidelity scalp hair synthesis.
Organic Open-cell Porous Structure Modeling
Lihao Tian, Lin Lu, Weikai Chen, Yang Xia, Charlie C. L. Wang and Wenping Wang
ACM Symposium on Computational Fabrication 2020
"controllable field-aligned porous structure modeling"
Lihao Tian, Lin Lu, Weikai Chen, Yang Xia, Charlie C. L. Wang and Wenping Wang
ACM Symposium on Computational Fabrication 2020
"controllable field-aligned porous structure modeling"
- paper
-
abstract
Open-cell porous structures are ubiquitous in nature and have been widely employed in practical applications. Additive manufacturing has enabled the fabrication of shapes with intricate interior structures; however, a computational method for representing and modeling general porous structures in organic shapes is missing in the literature. In this paper, we present a novel method for modeling organic and open-cell porous structures with porosities and pore anisotropies specified by users or stipulated by applications. We represent each pore as a transformed Gaussian kernel whose anisotropy is defined by a tensor field. The porous structure is modeled as a level surface of combined Gaussian kernels. We utilize an anisotropic particle system to distribute the Gaussian kernels concerning the input tensor field. The porous structure is then generated from the particle system by following the anisotropy specified by the input. We employ Morse-Smale complexes to identify the topological structure of the kernels and enforce pore connectivity. The resulting porous structure can be easily controlled using a set of parameters. We demonstrate our method on a set of 3D models whose tensor field is either predesigned or obtained from the mechanical analysis..
Learning to Infer Implicit Surfaces without 3D Supervision
Shichen Liu, Shunsuke Saito, Weikai Chen, Hao Li
NeurIPS 2019
"the first differentiable renderer for implicit field"
Shichen Liu, Shunsuke Saito, Weikai Chen, Hao Li
NeurIPS 2019
"the first differentiable renderer for implicit field"
- paper
-
abstract
Recent advances in 3D deep learning have shown that it is possible to train highly effective deep models for 3D shape generation, directly from 2D images. This is particularly interesting since the availability of 3D models is still limited compared to the massive amount of accessible 2D images, which is invaluable for training. The representation of 3D surfaces itself is a key factor for the quality and resolution of the 3D output. While explicit representations, such as point clouds and voxels, can span a wide range of shape variations, their resolutions are often limited. Mesh-based representations are more efficient but are limited by their ability to handle varying topologies. Implicit surfaces, however, can robustly handle complex shapes, topologies, and also provide flexible resolution control. We address the fundamental problem of learning implicit surfaces for shape inference without the need of 3D supervision. Despite their advantages, it remains nontrivial to (1) formulate a differentiable connection between implicit surfaces and their 2D renderings, which is needed for image-based supervision; and (2) ensure precise geometric properties and control, such as local smoothness. In particular, sampling implicit surfaces densely is also known to be a computationally demanding and very slow operation. To this end, we propose a novel ray-based field probing technique for efficient image-to-field supervision, as well as a general geometric regularizer for implicit surfaces, which provides natural shape priors in unconstrained regions. We demonstrate the effectiveness of our framework on the task of single-view image-based 3D shape digitization and show how we outperform state-of-the-art techniques both quantitatively and qualitatively.
Soft Rasterizer: A Differentiable Renderer for Image-based 3D Reasoning
Shichen Liu, Tianye Li, Weikai Chen, Hao Li
ICCV 2019 (Oral Presentation) - 3 Strong Accepts
"a truly differentiable renderer for rasterization-based rendering"
Shichen Liu, Tianye Li, Weikai Chen, Hao Li
ICCV 2019 (Oral Presentation) - 3 Strong Accepts
"a truly differentiable renderer for rasterization-based rendering"
- paper
- code
- old version
-
abstract
Rendering bridges the gap between 2D vision and 3D scenes by simulating the physical process of image formation. By inverting such renderer, one can think of a learning approach to infer 3D information from 2D images. However, standard graphics renderers involve a fundamental discretization step called rasterization, which prevents the rendering process to be differentiable, hence able to be learned. Unlike the state-of-the-art differentiable renderers, which only approximate the rendering gradient in the back propagation, we propose a truly differentiable rendering framework that is able to (1) directly render colorized mesh using differentiable functions and (2) back-propagate efficient supervision signals to mesh vertices and their attributes from various forms of image representations, including silhouette, shading and color images. The key to our framework is a novel formulation that views rendering as an aggregation function that fuses the probabilistic contributions of all mesh triangles with respect to the rendered pixels. Such formulation enables our framework to flow gradients to the occluded and far-range vertices, which cannot be achieved by the previous state-of-the-arts. We show that by using the proposed renderer, one can achieve significant improvement in 3D unsupervised single-view reconstruction both qualitatively and quantitatively. Experiments also demonstrate that our approach is able to handle the challenging tasks in image-based shape fitting, which remain nontrivial to existing differentiable renderers.
Learning Perspective Undistortion of Portraits
Yajie Zhao*, Zeng Huang*, Tianye Li, Weikai Chen, Chloe LeGendre, Xinglei Ren, Ari Shapiro, and Hao Li
ICCV 2019 (Oral Presentation)
"a method for recifying facial distortions in near-range portraits"
Yajie Zhao*, Zeng Huang*, Tianye Li, Weikai Chen, Chloe LeGendre, Xinglei Ren, Ari Shapiro, and Hao Li
ICCV 2019 (Oral Presentation)
"a method for recifying facial distortions in near-range portraits"
- paper
-
abstract
Near-range portrait photographs often contain perspective distortion artifacts that bias human perception and challenge both facial recognition and reconstruction techniques. We present the first deep learning based approach to remove such artifacts from unconstrained portraits. In contrast to the previous state-of-the-art approach, our method handles even portraits with extreme perspective distortion, as we avoid the inaccurate and error-prone step of first fitting a 3D face model. Instead, we predict a distortion correction flow map that encodes a per-pixel displacement that removes distortion artifacts when applied to the input image. Our method also automatically infers missingfacial features, i.e. occluded ears caused by strong perspective distortion, with coherent details. We demonstrate that our approach significantly outperforms the previous stateof-the-art both qualitatively and quantitatively, particularly for portraits with extreme perspective distortion or facial expressions. We further show that our technique benefits a number of fundamental tasks, significantly improving the accuracy of both face recognition and 3D reconstruction and enables a novel camera calibration technique from a single portrait. Moreover, we also build the first perspective portrait database with a large diversity in identities, expression and poses.
Deep Mesh Reconstruction from Single RGB Images via Topology Modification Networks
Junyi Pan, Xiaoguang Han, Weikai Chen, Jiapeng Tang and Kui Jia
ICCV 2019
"a single-view mesh reconstruction approach that can handle objects with arbitrary topologies"
Junyi Pan, Xiaoguang Han, Weikai Chen, Jiapeng Tang and Kui Jia
ICCV 2019
"a single-view mesh reconstruction approach that can handle objects with arbitrary topologies"
- paper
- code
-
abstract
Reconstructing the 3D mesh of a general object from a single image is now possible thanks to the latest advances of deep learning technologies. However, due to the nontrivial difficulty of generating a feasible mesh structure, the state-of-the-art approaches~\cite{wang2018pixel2mesh,kanazawa2018learning} often simplify the problem by learning the displacements of a template mesh that deforms it to the target surface.Though reconstructing a 3D shape with complex topo logy can be achieved by deforming multiple mesh patches, it remains difficult to stitch the results to ensure a high meshing quality. In this paper, we present an end-to-end single-view mesh reconstruction framework that is able to generate high-quality meshes with complex topology from a single genus-0 template mesh. The key to our approach is a novel progressive shaping framework that alternates between shape deformation and topology modifying. While a deformation network predicts the per-vertex translations that reduce the gap between the reconstructed mesh and the ground truth, a novel topology modification network is employed to prune the error-prone faces and refine the boundary conditions, enabling the evolution of topology. By iterating over the two procedures, one can progressively modify the mesh topology while achieving higher reconstruction accuracy. Extensive experiments demonstrate that our approach significantly outperforms the current state-of-the-art methods both qualitatively and quantitatively, especially for the shapes with complex topology.
HairBrush for Immersive Data-Driven Hair Modeling
Jun Xing, Koki Nagano, Weikai Chen, Haotian Xu, Li-Yi Wei, Jingwan Lu, Byungmoon Kim, Yajie Zhao, Hao Li
UIST 2019
"an immersive hair modeling tool in VR"
Jun Xing, Koki Nagano, Weikai Chen, Haotian Xu, Li-Yi Wei, Jingwan Lu, Byungmoon Kim, Yajie Zhao, Hao Li
UIST 2019
"an immersive hair modeling tool in VR"
- project page
- paper
-
abstract
While hair is an essential component of virtual humans, it is also one of the most challenging and time-consuming digital assets to create. Existing automatic techniques lack the generality and flexibility for users to create the exact intended hairstyles. Meanwhile, manual authoring interfaces often require considerable skills and experiences from character modelers, and are difficult to navigate for intricate 3D hair structures. We propose an interactive hair modeling system that can help create complex hairstyles that would otherwise take weeks or months with existing tools. Modelers, including novice users, can focus on the overall intended hairstyles and local hair deformations, as our system intelligently suggests the desired hair parts. Our method combines the flexibility of manual authoring and the convenience of data-driven automation. Since hair contains intricate 3D structures such as buns, knots, and strands, they are inherently challenging to create from scratch using traditional 2D interfaces. Our system provides a new 3D hair authoring interface for immersive interaction in virtual reality (VR). We use a strip-based representation, which is commonly adopted in real-time games due to rendering efficiency and modeling flexibility. The output strips can be converted to other hair formats such as strands. Users can draw high-level guide strips, from which our system predicts the most plausible hairstyles in the dataset via a trained deep neural network. Each hairstyle in our dataset is composed of multiple variations, serving as blendshapes to fit the user drawings via global blending and local deformation. The fitted hair models are visualized as interactive suggestions, that the user can select, modify, or ignore. We conducted a user study to confirm that our system can significantly reduce manual labor while improve the output quality for modeling a variety of hairstyles that are challenging to create using existing techniques.
SiCloPe: Silhouette-Based Clothed People
Ryota Natsume, Shunsuke Saito, Zeng Huang, Weikai Chen, Chongyang Ma, Hao Li, Shigeo Morishima
CVPR 2019 (Oral Presentation) - CVPR Best Paper Finalists
"single-view based clothed human reconstruction"
Ryota Natsume, Shunsuke Saito, Zeng Huang, Weikai Chen, Chongyang Ma, Hao Li, Shigeo Morishima
CVPR 2019 (Oral Presentation) - CVPR Best Paper Finalists
"single-view based clothed human reconstruction"
- paper
-
abstract
We introduce a new silhouette-based representation for modeling clothed human bodies using deep generative models. Our method can reconstruct a complete and textured 3D model of a person wearing clothes from a single input picture. Inspired by the visual hull algorithm, our implicit representation uses 2D silhouettes and 3D joints of a body pose to describe the immense shape complexity and variations of clothed people. Given a segmented 2D silhouette of a person and its inferred 3D joints from the input picture, we first synthesize consistent silhouettes from novel view points around the subject. The synthesized silhouettes, which are the most consistent with the input segmentation are fed into a deep visual hull algorithm for robust 3D shape prediction. We then infer the texture of the subject's back view using the frontal image and segmentation mask as input to a conditional generative adversarial network. Our experiments demonstrate that our silhouette-based model is an effective representation and the appearance of the back view can be predicted reliably using an image-to-image translation network. While classic methods based on parametric models often fail for single-view images of subjects with challenging clothing, our approach can still produce successful results, which are comparable to those obtained from multi-view input.
Mask-off: Synthesizing Face Images in the Presence of Head-mounted Displays
Yajie Zhao, Qingguo Xu, Weikai Chen, Jun Xing, Chao Du, Xinyu Huang, Ruigang Yang
IEEE VR 2019
"enabling face-to-face communication in the presence of head-mounted display"
Yajie Zhao, Qingguo Xu, Weikai Chen, Jun Xing, Chao Du, Xinyu Huang, Ruigang Yang
IEEE VR 2019
"enabling face-to-face communication in the presence of head-mounted display"
- paper
-
abstract
Wearable VR/AR devices provide users with fully immersive experience in a virtual environment, enabling possibilities to reshape the forms of entertainment and telepresence. While the body language is a crucial element in effective communication, wearing a head-mounted display (HMD) could severely hinder the eye contact and block facial expressions. We present a novel headset removal technique that enables high-quality occlusion-free communication in virtual environment. In particular, our solution synthesizes photoreal faces in the occluded region with faithful reconstruction of facial expressions and eye movements. Towards this goal, we develop a novel capture setup that consists of two near-infrared (NIR) cameras inside the HMD for eye capturing and one external RGB camera for recording visible face regions. To enable realistic face synthesis with consistent illuminations, we propose a data-driven approach to fuse the narrow-field-of-view NIR images with the RGB image captured from the external camera. In addition, to generate photorealistic eyes, a dedicated algorithm is proposed to colorize the NIR eye images and further rectify the color distortion caused by the non-linear mapping of IR light sensitivity. Experimental results demonstrate that our framework is capable to synthesize high-fidelity unoccluded facial images with accurate tracking of head motion, facial expression and eye movement.
Deep Volumetric Video From Very Sparse Multi-View Performance Capture
Zeng Huang, Tianye Li, Weikai Chen, Yajie Zhao, Jun Xing, Chloe LeGendre, Linjie Luo, Chongyang Ma, Hao Li
ECCV 2018
"volumetric body reconstruction from highly sparse views"
Zeng Huang, Tianye Li, Weikai Chen, Yajie Zhao, Jun Xing, Chloe LeGendre, Linjie Luo, Chongyang Ma, Hao Li
ECCV 2018
"volumetric body reconstruction from highly sparse views"
- paper
- video
-
abstract
We present a deep learning based volumetric approach for performance capture using a passive and highly sparse multi-view capture system. State-of-the-art performance capture systems require either pre-scanned actors, large number of cameras or active sensors. In this work, we focus on the task of template-free, per-frame 3D surface reconstruction from as few as three RGB sensors, for which conventional visual hull or multi-view stereo methods fail to generate plausible results.We introduce a novel multi-view Convolutional Neural Network (CNN) that maps 2D images to a 3D volumetric field and we use this field to encode the probabilistic distribution of surface points of the captured subject. By querying the resulting field, we can instantiate the clothed human body at arbitrary resolutions. Our approach scales to different numbers of input images, which yield increased reconstruction quality when more views are used. Although only trained on synthetic data, our network can generalize to handle real footage from body performance capture. Our method is suitable for high-quality low-cost full body volumetric capture solutions, which are gaining popularity for VR and AR content creation. Experimental results demonstrate that our method is significantly more robust and accurate than existing techniques when only very sparse views are available.
- suppl.
HairNet: Single-View Hair Reconstruction using Convolutional Neural Networks
Yi Zhou, Liwen Hu, Jun Xing, Weikai Chen, Han-Wei Kung, Xin Tong, Hao Li
ECCV 2018
"deep learning based 3D hair reconstruction from a single image"
Yi Zhou, Liwen Hu, Jun Xing, Weikai Chen, Han-Wei Kung, Xin Tong, Hao Li
ECCV 2018
"deep learning based 3D hair reconstruction from a single image"
- paper
- video
-
abstract
We introduce a deep learning-based method to generate full 3D hair geometry from an unconstrained image. Our method can recover local strand details and has real-time performance. State-of-the-art hair modeling techniques rely on large hairstyle collections for nearest neighbor retrieval and then perform ad-hoc refinement. Our deep learning approach, in contrast, is highly efficient in storage and can run 1000 times faster while generating hair with 30K strands. The convolutional neural network takes the 2D orientation field of a hair image as input and generates strand features that are evenly distributed on the parameterized 2D scalp. We introduce a collision loss to synthesize more plausible hairstyles, and the visibility of each strand is also used as a weight term to improve the reconstruction accuracy. The encoder-decoder architecture of our network naturally provides a compact and continuous representation for hairstyles, which allows us to interpolate naturally between hairstyles. We use a large set of rendered synthetic hair models to train our network. Our method scales to real images because an intermediate 2D orientation field, automatically calculated from the real image, factors out the difference between synthetic and real hairs. We demonstrate the effectiveness and robustness of our method on a wide range of challenging real Internet pictures and show reconstructed hair sequences from videos.
- MIT Tech Review
- Nvidia Review
High-Fidelity Facial Reflectance and Geometry Inference From an Unconstrained Image
Shugo Yamaguchi*, Shunsuke Saito*, Koki Nagano, Yajie Zhao, Weikai Chen, Shigeo Morishima, Hao Li
SIGGRAPH 2018 (ACM Transactions on Graphics)
"inference of complete face reflectance maps from a single unconstrained image"
Shugo Yamaguchi*, Shunsuke Saito*, Koki Nagano, Yajie Zhao, Weikai Chen, Shigeo Morishima, Hao Li
SIGGRAPH 2018 (ACM Transactions on Graphics)
"inference of complete face reflectance maps from a single unconstrained image"
- paper
- video
-
abstract
We present a deep learning-based technique to infer high-quality facial reflectance and geometry given a single unconstrained image of the subject, which may contain partial occlusions and arbitrary illumination conditions. The reconstructed high-resolution textures, which are generated in only a few seconds, include high-resolution skin surface reflectance maps, representing both the diffuse and specular albedo, and medium- and highfrequency displacement maps, thereby allowing us to render compelling digital avatars under novel lighting conditions. To extract this data, we train our deep neural networks with a high-quality skin reflectance and geometry database created with a state-of-the-art multi-view photometric stereo system using polarized gradient illumination. Given the raw facial texture map extracted from the input image, our neural networks synthesize complete reflectance and displacement maps, as well as complete missing regions caused by occlusions. The completed textures exhibit consistent quality throughout the face due to our network architecture, which propagates texture features from the visible region, resulting in high-fidelity details that are consistent with those seen in visible regions. We describe how this highly underconstrained problem is made tractable by dividing the full inference into smaller tasks, which are addressed by dedicated neural networks. We demonstrate the effectiveness of our network design with robust texture completion from images of faces that are largely occluded. With the inferred reflectance and geometry data, we demonstrate the rendering of high-fidelity 3D avatars from a variety of subjects captured under different lighting conditions. In addition, we perform evaluations demonstrating that our method can infer plausible facial reflectance and geometric details comparable to those obtained from high-end capture devices, and outperform alternative approaches that require only a single unconstrained input image.
- suppl.
Mesoscopic Facial Geometry inference Using Deep Neural Networks
Loc Huynh, Weikai Chen, Shunsuke Saito, Jun Xing, Koki Nagano, Andrew Jones, Hao Li, Paul Debevec
CVPR 2018 (Spotlight Presentation)
"pore-level facial geometry inference from a single image"
Loc Huynh, Weikai Chen, Shunsuke Saito, Jun Xing, Koki Nagano, Andrew Jones, Hao Li, Paul Debevec
CVPR 2018 (Spotlight Presentation)
"pore-level facial geometry inference from a single image"
- paper
-
abstract
We present a learning-based approach for synthesizing facial geometry at medium and fine scales from diffusely-lit facial texture maps. When applied to an image sequence, the synthesized detail is temporally coherent. Unlike current state-of-the-art methods [17, 5], which assume ”dark is deep”, our model is trained with measured facial detail collected using polarized gradient illumination in a Light Stage [20]. This enables us to produce plausible facial detail across the entire face, including where previous approaches may incorrectly interpret dark features as concavities such as at moles, hair stubble, and occluded pores. Instead of directly inferring 3D geometry, we propose to encode fine details in high-resolution displacement maps which are learned through a hybrid network adopting the state-of-the-art image-to-image translation network [29] and super resolution network [43]. To effectively capture geometric detail at both mid- and high frequencies, we factorize the learning into two separate sub-networks, enabling the full range of facial detail to be modeled. Results from our learning-based approach compare favorably with a high-quality active facial scanhening technique, and require only a single passive lighting condition without a complex scanning setup.
Identity Preserving Face Completion for Large Ocular Region Occlusion
Yajie Zhao, Weikai Chen, Jun Xing, Xiaoming Li, Zach Bessinger, Fuchang Liu, Wangmeng Zuo, Ruigang Yang
BMVC 2018
"identity-preserved face inpainting for large occlusions"
Yajie Zhao, Weikai Chen, Jun Xing, Xiaoming Li, Zach Bessinger, Fuchang Liu, Wangmeng Zuo, Ruigang Yang
BMVC 2018
"identity-preserved face inpainting for large occlusions"
- paper
-
abstract
We present a novel deep learning approach to synthesize complete face images in the presence of large ocular region occlusions. This is motivated by recent surge of VR/AR displays that hinder face-to-face communications. Different from the state-of-the-art face inpainting methods that have no control over the synthesized content and can only handle frontal face pose, our approach can faithfully recover the missing content under various head poses while preserving the identity. At the core of our method is a novel generative etwork with dedicated constraints to regularize the synthesis process. To preserve the identity, our network takes an arbitrary occlusion-free image of the target identity to infer the missing content, and its high-level CNN features as an identity prior to regularize the searching space of generator. Since the input reference image may have a different pose, a pose map and a novel pose discriminator are further adopted to supervise the learning of implicit pose transformations. Our method is capable of generating coherent facial inpainting with consistent identity over videos with large variations of head motions. Experiments on both synthesized and real data demonstrate that our method greatly outperforms the state-of-the-art methods in terms of both synthesis quality and robustness.
Deep RBFNet: Point Cloud Feature Learning using Radial Basis Functions
Weikai Chen, Xiaoguang Han, Guanbin Li, Chao Chen, Jun Xing, Yajie Zhao, Hao Li
arXiv 2018
"deep point cloud feature based on radial basis functions"
Weikai Chen, Xiaoguang Han, Guanbin Li, Chao Chen, Jun Xing, Yajie Zhao, Hao Li
arXiv 2018
"deep point cloud feature based on radial basis functions"
- paper
-
abstract
Three-dimensional object recognition has recently achieved great progress thanks to the development of effective point cloud-based learning frameworks, such as PointNet and its extensions. However, existing methods rely heavily on fully connected layers, which introduce a significant amount of parameters, making the network harder to train and prone to overfitting problems. In this paper, we propose a simple yet effective framework for point set feature learning by leveraging a nonlinear activation layer encoded by Radial Basis Function (RBF) kernels. Unlike PointNet variants, that fail to recognize local point patterns, our approach explicitly models the spatial distribution of point clouds by aggregating features from sparsely distributed RBF kernels. A typical RBF kernel, e.g. Gaussian function, naturally penalizes long-distance response and is only activated by neighboring points. Such localized response generates highly discriminative features given different point distributions. In addition, our framework allows the joint optimization of kernel distribution and its receptive field, automatically evolving kernel configurations in an end-to-end manner. We demonstrate that the proposed network with a single RBF layer can outperform the state-of-the-art Pointnet++ in terms of classification accuracy for 3D object recognition tasks. Moreover, the introduction of nonlinear mappings significantly reduces the number of network parameters and computational cost, enabling significantly faster training and a deployable point cloud recognition solution on portable devices with limited resources.
Fabricable Tile Decors
Weikai Chen*, Yuexin Ma*, Sylvain Lefebvre, Shiqing Xin, Jonàs Martínez and Wenping Wang
SIGGRAPH Asia 2017 (ACM Transactions on Graphics)
"a *magic* way to fabricate curved surfaces flatly"
Weikai Chen*, Yuexin Ma*, Sylvain Lefebvre, Shiqing Xin, Jonàs Martínez and Wenping Wang
SIGGRAPH Asia 2017 (ACM Transactions on Graphics)
"a *magic* way to fabricate curved surfaces flatly"
- project page
- paper
- video
-
abstract
Recent advances in 3D printing have made it easier to manufacture customized objects by ordinary users in an affordable manner, and therefore spurred high demand for more accessible methods for designing and fabricating 3D objects of various shapes and functionalities. In this paper we present a novel approach to model and fabricate surface-like objects composed of connected tiles, which can be used as objects in daily life, such as ornaments, covers, shades or handbags. Our method is designed to maximize the efciency and ease of fabrication. Given a base surface and a set of tile elements as user input, our method generates a tight packing of connected tiles on the surface. We apply an efcient and tailored optimization scheme to pack the tiles on the base surface with fabrication constraints. Then, to facilitate the fabrication process, we use a novel method based on minimal spanning tree to decompose the set of connected tiles into several connected patches. Each patch is articulated and can be developed into a plane. This allows printing with an inexpensive FDM printing process without requiring any supporting structures, which are often troublesome to remove. Finally, the separately printed patches are reassembled to form the fnal physical object, a shell surface composed of connected user-specifed tiles that take the shape of the input base surface. We demonstrate the utility of our method by modeling and fabricating a variety of objects, from simple decorative spheres to moderately complex surfaces, such as a handbag and a teddy bear. Several user controls are available, to distribute different type of tiles over the surface and locally change their scales and orientations
Tensor Field Design in Volumes
Jonathan Palacios, Lawrence Roy, Prashant Kumar, Chen-Yuan Hsu, Weikai Chen, Chongyang Ma, Li-Yi Wei, Eugene Zhang
SIGGRAPH Asia 2017 (ACM Transactions on Graphics)
"the first framework to design and edit 3D tensors"
Jonathan Palacios, Lawrence Roy, Prashant Kumar, Chen-Yuan Hsu, Weikai Chen, Chongyang Ma, Li-Yi Wei, Eugene Zhang
SIGGRAPH Asia 2017 (ACM Transactions on Graphics)
"the first framework to design and edit 3D tensors"
- project page
- paper
- video
-
abstract
3D tensor field design is important in several graphics applications such as procedural noise, solid texturing, and geometry synthesis. Different fields can lead to different visual effects. The topology of a tensor !eld, such as degenerate tensors, can cause artifacts in these applications. Existing 2D tensor field design systems cannot be used to handle the topology of a 3D tensor field. In this paper, we present to our knowledge the first 3D tensor !eld design system. At the core of our system is the ability to edit the topology of tensor !elds. We demonstrate the power of our design system with applications in solid texturing and geometry synthesis.
By Example Synthesis of Three-Dimensional Porous Materials
Hui Zhang, Weikai Chen, Bin Wang and Wenping Wang
GMP 2017
"example-based 3D porous structure synthesis"
Hui Zhang, Weikai Chen, Bin Wang and Wenping Wang
GMP 2017
"example-based 3D porous structure synthesis"
- paper
-
abstract
Porous materials are ubiquitous in nature and are used for many applications. However, there is still a lack of computational methods for generating and modeling complex porous structures. While conventional texture synthesis methods succeed in synthesizing solid texture based on a 2D input, to generate a 3D structure that visually matches a given 3D exemplar remains an open question. We present the first framework that can synthesize porous material that is structurally consistent to input 3D exemplar. In our framework, the 2D texture optimization method is extended built upon 3D neighborhood. An adaptive weighted mechanism method is proposed to reduce blurring and accelerate the convergence speed. Moreover, a connectivity pruning algorithm is performed as post-processing to prune spurious branches. Experimental results demonstrate that our method can preserve both the structural continuity and material descriptors of input exemplar while maintain visual similarity with input structure.
Synthesis of Filigrees for Digital Fabrication
Weikai Chen, Xiaolong Zhang, Shiqing Xin, Yang Xia , Sylvain Lefebvre and Wenping Wang
SIGGRAPH 2016 (ACM Transactions on Graphics)
"how to make example-based 3D jewelry"
Weikai Chen, Xiaolong Zhang, Shiqing Xin, Yang Xia , Sylvain Lefebvre and Wenping Wang
SIGGRAPH 2016 (ACM Transactions on Graphics)
"how to make example-based 3D jewelry"
- project page
- paper
- video
-
abstract
Filigrees are thin patterns found in jewelry, ornaments and lace fabrics. They are often formed of repeated base elements manually composed into larger, delicate patterns. Digital fabrication simplifies the process of turning a virtual model of a filigree into a physical object. However, designing a virtual model of a filigree remains a time consuming and challenging task. The difficulty lies in tightly packing together the base elements while covering a target surface. In addition, the filigree has to be well connected and sufficiently robust to be fabricated. We propose a novel approach automating this task. Our technique covers a target surface with a set of input base elements, forming a filigree strong enough to be fabricated. We exploit two properties of filigrees to make this possible. First, as filigrees form delicate traceries they are well captured by their skeleton. This affords for a simpler definition of operators such as matching and deformation. Second, instead of seeking for a perfect packing of the base elements we relax the problem by allowing appearance preserving partial overlaps. We optimize a filigree by a stochastic search, further improved by a novel boosting algorithm that records and reuses good configurations discovered during the process. We illustrate our technique on a number of challenging examples reproducing filigrees on large objects, which we manufacture by 3D printing. Our technique affords for several user controls, such as the scale and orientation of the elements.
- two-minute paper
Tensor Field Design in Volumes
Jonathan Palacios, Chongyang Ma, Weikai Chen, Li-Yi Wei and Eugene Zhang
SIGGRAPH Asia 2016 Technical Briefs
"a lightweight 3D tensor designing and editing system"
Jonathan Palacios, Chongyang Ma, Weikai Chen, Li-Yi Wei and Eugene Zhang
SIGGRAPH Asia 2016 Technical Briefs
"a lightweight 3D tensor designing and editing system"
- project page
- paper
-
abstract
The design of 3D tensor fields is important in several graphics applications such as procedural noise, solid texturing, and geometry synthesis. Different fields can lead to different visual effects. The topology of a tensor field, such as degenerate tensors, can cause artifacts in these applications. Existing 2D tensor field design systems cannot handle the topology of 3D tensor fields. We present, to our best knowledge, the first 3D tensor field design system. At the core of our system is the ability to specify and control the type, number, location, shape, and connectivity of degenerate tensors. To enable such capability, we have made a number of observations of tensor field topology that were previously unreported. We demonstrate applications of our method in volumetric synthesis of solid and geometry texture as well as anisotropic Gabor noise.
Second-order differential based matching pursuit method for compressive sensing signal recovery
Weikai Chen, Yunhui Chen
WCSP 2012
"all about signal compression"
Weikai Chen, Yunhui Chen
WCSP 2012
"all about signal compression"
A Compressive Sensing Method for Estimating Doubly-Selective Sparse Channels in OFDM Systems
Kaihua Liu, Weikai Chen (corresponding author), Yongtao Ma
Journal of Tianjin University
"channel estimation for OFDM system"
Kaihua Liu, Weikai Chen (corresponding author), Yongtao Ma
Journal of Tianjin University
"channel estimation for OFDM system"
Ph.D. Dissertation
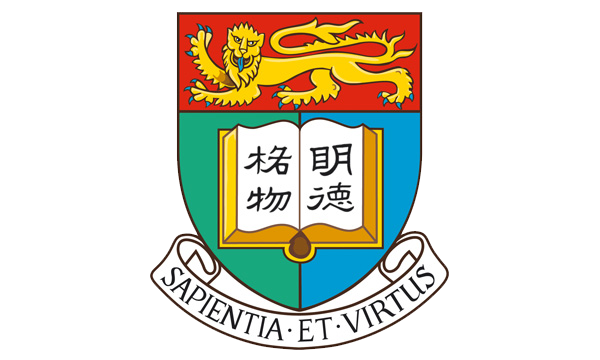
Synthesizing patterned surfaces for 3D printing
Weikai Chen
The University of Hong Kong, 2017
"basically a concatenation of my first two SIGGRAPH/SIGGRAPH Asia papers"
Weikai Chen
The University of Hong Kong, 2017
"basically a concatenation of my first two SIGGRAPH/SIGGRAPH Asia papers"
- thesis
-
abstract
Recent years have witnessed the advancement of 3D printing in fabricating objects with sophisticated and highly-customized geometries. The print services are now widely available through online orders, home printers and local FabLabs. Nevertheless, it remains difficult for most users to create interesting objects, even more so when the intended design has complex geometry details. To circumvent this issue, in this thesis we present approaches to automate the task of designing and fabricating artistic patterned surfaces.
We firstly present a novel approach to synthesize fabricable filigrees over target surfaces. As thin patterns widely found in jewelry, ornaments and lace fabrics, filigrees are often manually designed by composing repeated base elements. Our technique aims to automate this challenging task. Our technique covers a target surface with a set of input base elements, forming a filigree strong enough to be fabricated. We leverage the fact that as traceries, filigrees can be well captured by their skeletons. This affords for novel energy function that measures the matching quality between base elements. In addition, instead of seeking for a perfect packing of base elements, we relax the problem by allowing appearance-preserving partial overlaps. The formulation is optimized by a stochastic search, which is further improved by a boosting step that records and reuses good configurations discovered during process. Our technique affords for multi-class synthesis and several user controls, such as scale and orientation of the elements.
Second, we extend the method to generate complex – yet easy to print -- tile decorations. The user only provides base surface and a set of tiles. Our algorithm automatically decorates the base surface with the tiles. However, rather than being simple decals, the tiles \textit{become} the final object, producing shell-like surfaces that can be used as ornaments, covers, shades and even handbags. Our technique is designed to maximize print efficiency: the results are printed as independent flat patches that are articulated sets of tiles. The patches could be assembled into the final surface through the use of snap-fit connectors. Our approach proceeds in three steps. First, a dedicated packing algorithm is proposed to compute a tile layout while taking into account fabrication constraints, in particular ensuring hinges can be inserted between neighboring tiles. A second step extracts the patches to be printed and folded, while the third step optimizes the location of snap-fit connectors. Our technique works on a variety of objects, from simple decorative spheres to moderately complex shapes. -
bibtex
@phdthesis{chen2017synthesizing, title={Synthesizing patterned surfaces for 3D printing}, author={Chen, Weikai}, journal={HKU Theses Online (HKUTO)}, year={2017}, publisher={The University of Hong Kong (Pokfulam, Hong Kong)} }